What is descriptive analytics?
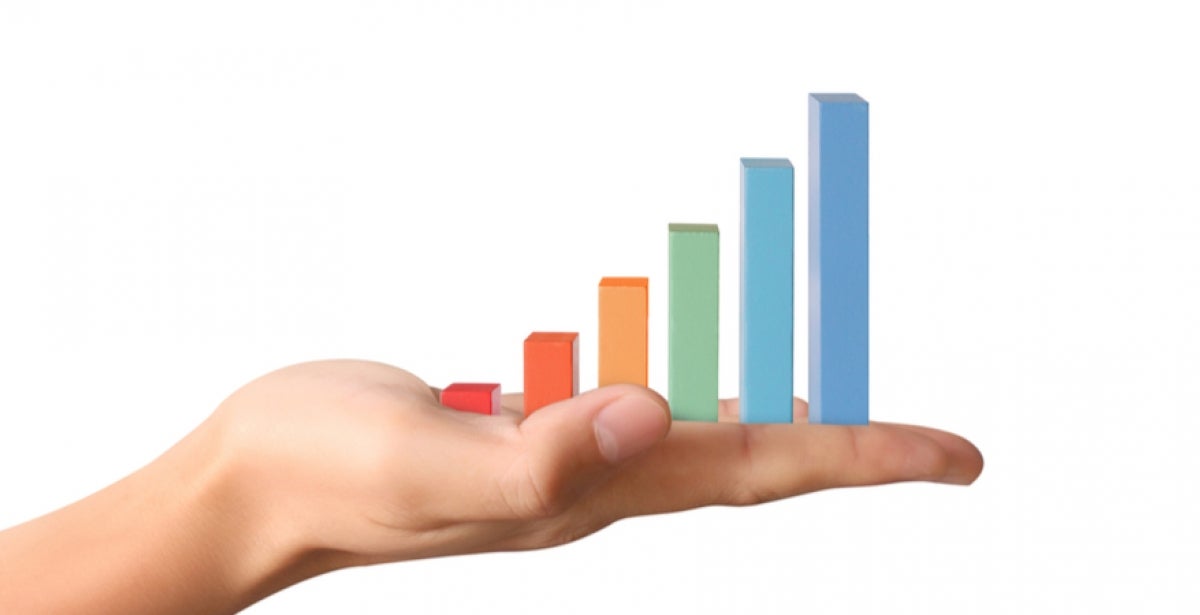
The magic of data, most believe, is in its ability to help us to predict the future. Rather than always looking backwards, data allows us to finally look forward, to plot the most likely pathway to success, In other words, data is all about predictive analytics.
But increasingly, business managers and data scientists are realising there is enormous value in using data to analyse past events. After all, until we clearly understand past patterns and trends, how can we possibly predict what might happen in the future?
The art of interpreting historical data to understand changes that have occurred, or trends that have developed or decisions customers have made, is known as ‘descriptive analytics’. It is developing into a powerful discipline of its own in the ever-evolving data science arena.
So how does descriptive analytics work, and what is its core purpose or power?
Some define descriptive analytics as ‘What happened?’. To explain this, if predictive analytics is about ‘What could happen?’ and prescriptive analytics is ‘What should we do?’, descriptive analytics is really about trying to understand what happened.
It might involve looking at a very specific event, a trend that developed, a client reaction or a reason that customers chose the blue widget over the red one.
How does descriptive analytics work?
In any organisation, no matter how small or large, there is an enormous amount going on in and around the business. If an event occurred, such as a sudden influx of customers, it can be very difficult to pinpoint the exact reason for that good fortune.
Was it a new product launch, the training of a team of customer service agents, a competitor going out of business, or a particular marketing campaign? Or, more likely, was the influx driven by a unique mix of a number of events? And if a specific marketing campaign made such a noticeable difference, what exactly was it about the campaign that caused such success when others did not?
Once the reason or reasons for the event are pinpointed, they can be replicated at other times, with other cohorts of customers and in other markets, in order to experience the same success.
This is where descriptive analytics comes in. And, of course, it’s not just about business. The same data science can be used in other applications, such as investigating a successful medical treatment for childhood cancer or developing a better understanding of natural disaster events.
Data scientists begin with a specific question or set of questions (‘Why did our sales spike in May?’, ‘Why did we lose 15 per cent market share in 2018?’, etc.), then gather large sets of raw data around those questions and events. This process involves figuring out which metrics are important to answer the question and, just as importantly, which are not.
During this process, the correct data is identified and can then be gathered and prepared before the analyses are run.
Descriptive analytics experts then separate that data into various component parts in order to overlay different sets over one another. With enough historical data and enough parsing, patterns emerge that might otherwise be impossible to identify.
The information that results from the descriptive analytics process is typically fairly basic and easy to understand, and it has to be. After all, it is intended to support business decisions by people across the organisation, so it must be easily digestible – see the section on data visualisation.
This information will also form the foundation from which further analytics processes are launched.
Descriptive analytics creates the bricks with which the predictive analytics processes – the ‘What could happen in the future?’ and ‘What is our best next move?’ – are built.
Data visualisation
An important part of descriptive analytics is data visualisation. This is the presentation of the results of the descriptive analytics process in a way that can be easily understood by people across the organisation, from marketing to finance, and from HR to customer service.
There are few rules, but plenty of tools, for data visualisation. The best solution is the one that is most easily understood by decision-makers in the organisation.
Today’s data visualisation software packages help data scientists to create bar charts and pie graphs, infographics and heat maps, as well as interactive visualisation tools that include dials or drop-downs that allow the user to see the changes that result from different inputs.
Why is this visualisation so important? Decision making based on data that has not been properly understood or digested will, by its very nature, lead to incorrect or inferior decisions. If the data is not fully and clearly understood, the resulting decisions will be made in an environment of ambiguity or ignorance.
In order to assist with ongoing decision making, for example, dashboards can be set up that are updated with real-time information, giving those within an organisation the opportunity to track their success metrics and fine tune the KPIs that are intended to continue to drive performance.
Good data visualisation software is not the answer to every business manager’s wishes. It is not a silver bullet. Training of end users around the agreed meaning and purpose of the data is still essential, as is a deep understanding amongst those end users of the roles and challenges of those around them.
Consensus around the meaning of the data and its relation to the agreed organisational strategy is vital. In other words, all decision makers must be on the same page and must share the same understanding of the meaning of the data before good decisions can be made.
This leads to the final point around descriptive analytics. Professionals in this field become far more valuable to an organisation or industry when they invest in their further education, through courses such as the Master of Analytics. Such postgraduate study not only introduces candidates to a deeper level of knowledge in the field of analytics, but also offers them vital insight into the management challenges of organisations, and of other departments within those organisations.
Find out more about how to become an expert in descriptive analytics by studying a Master of Analytics. Get in touch with our Enrolment advisors on 1300 974 990.