How to become a data scientist
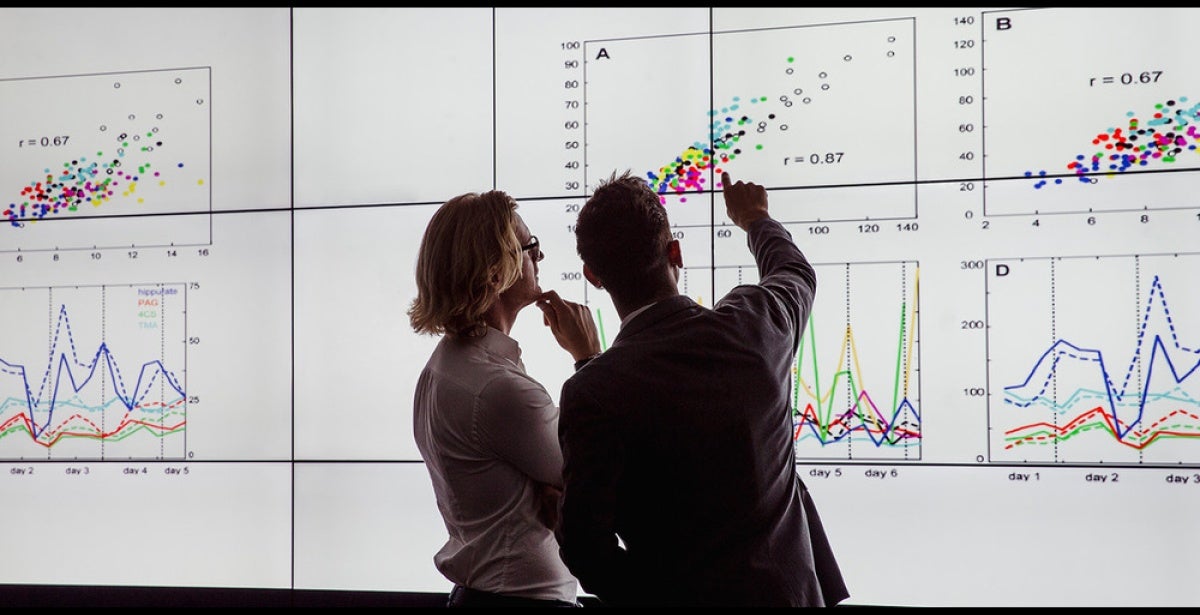
Data science is a fast-growing profession that is making an enormous impact on every corner of the economy. From business, medicine and entertainment to academia and politics, the vast number of technologies and services that have been transformed by data science illustrates the power of the discipline. And as more organisations seek to leverage this power, the demand for data scientists with the right education is stronger than ever.
Yet, high demand for data scientists does not mean that the profession is easy to enter. Despite being a highly-paid profession, data science positions are heavily short-staffed, which speaks to the complexities of entering the field. Learning how to become a data scientist can be challenging, as there’s no single defined path. However, with the right roadmap, it’s a journey that anyone can complete successfully.
Why become a data scientist?
There’s no doubt that data scientist salaries are high and that the position is in-demand and growing. And there are a number of other reasons aspiring data scientists should be interested in the field.
1. It’s interesting, impactful work
While data scientists focus their work on data, what they’re researching, analysing and interpreting is human behaviour. For example, data scientists may create a chatbot to help better understand customers online, or they may discover important and life-changing health or climate change trends. The work that data scientists do is hugely impactful and can both identify human behaviour and change it.
2. It helps you become a data-driven decision-maker
Increasingly, people are using data to make informed decisions, from reading online reviews to deciding which cafe to visit, to using expense tracking apps to increase their savings.
Data is everywhere. Becoming a data scientist helps you make better decisions based on relevant information. Analysing and understanding data is a crucial skill, in work and life.
3. You’ll gain a diverse array of skills
Every day, data scientists use a wide array of skills, such as analytics, predictive modelling, coding, statistics and data visualisation and storytelling.
They also hone their written and oral skills as they present their analysis to stakeholders.
Prerequisites for success
Becoming a data scientist has no formal prerequisites. While a background in a discipline such as statistics can be advantageous, the skills necessary to become a data scientist can be learned by practising data science tasks and learning about the current data and technology landscape.
How to become a data scientist: 4 steps
Although there is no single path to becoming a data scientist, these steps can help you enter the field.
Step 1: Get started with data science informal learning
Data science is a complex field and the best way to prepare yourself for future formal studies in the field is to start learning about it yourself beforehand. By doing so, you can go into your formal studies with specific goals and an understanding of what to expect moving forward.
Individuals can start learning about data science in various ways. These include the following:
- Blogs such as Towards Data Science offer a fantastic overview of many different data science concepts.
- Websites such as Data Science Central provide industry news and the latest trends.
- Introductions to basic data science (many of these materials are available for free online) make it easy to become familiar with the various coding languages that are used in the field.
Gaining familiarity with data science blogs, articles and learning resources is the best way for aspiring data scientists to learn about the various paths available to them.
During this initial exploratory process, beginner data scientists should explore the subjects that they find most interesting further. Data scientists network with each other by sharing their work, so any subjects that pique your interest will have ample amounts of further reading material available.
Exploring data science in this manner not only increases familiarity with the field and its subdisciplines but also provides an excellent opportunity to identify the off- and online locations where data science conversations are happening and to learn about leading figures in the field. By paying attention to these conversations, aspiring data scientists can learn the norms of the field and discover opportunities that they might otherwise not know to look for.
Step 2: The data science networking process
The lack of a defined road map for becoming a data scientist makes networking an essential part of entering the field. Networking allows aspiring data scientists to learn from people who’ve already ‘made it’, make connections with fellow learners and begin establishing a profile in the data science community. These connections are important to discovering opportunities to apply for data science positions or take on other relevant roles.
LinkedIn is a useful networking website; however, many other sites also offer access to specialised data science conversations. These include the following:
- Websites such as r/datascience provide access to a data science peer group.
- Websites such as Stack Overflow offer anyone the opportunity to ask questions about how to perform specific data science tasks. Many of these questions are regularly answered by leading voices in the field.
- Specialised niche data science groups that meet up online and in person are accessible via niche networking websites such as Meetup.
Step 3: Start your own data science projects
One of the best ways to become a data scientist and establish yourself is to gain experience in the field and through projects. Through time spent networking and exploring the field, you’ll come across a variety of simple data science projects that can inspire your own interests. Many aspiring data scientists begin their careers by experimenting with data visualisations, such as those found at r/DataIsBeautiful.
Experimenting with different types of data science helps you identify areas you might want to specialise in. The process of learning how to start an independent data science project is also valuable preparation for future professional work and results in a portfolio of work to show to potential employers. Data science relies very heavily on creative problem solving and demonstrating your ability to complete a project independently is important to gaining a foothold in the field.
Building a portfolio of independent projects is important to networking because it provides you with the opportunity to show your peers what you can do. For example, new attendees to data science Meetups and other networking events often share access to their data science blogs or GitHub repositories when they introduce themselves. Your beginner data science project don’t have to be complex to provide a valuable demonstration of your skills and many resources suggest specific projects for beginners.
As data scientists build their skills, it can be useful for them to participate in competitive projects. Projects that have a competitive element include the following:
- Kaggle offers an ongoing series of free data science competitions that aspiring and professional data scientists can use to develop their skills. Because these challenges are ranked, they’re excellent for individuals to monitor how their skills develop over time.
- The International Data Analysis Olympiad (IDAO) is a competition that’s open to everyone, including students at any level, as well as new data scientists. The competition helps data scientists sharpen their skills so they can fill industry demand.
- Topcoder offers many different challenges for up-and-coming data scientists and sometimes also offers prizes for the winners. Competitions focus on data science, design, competitive programming and much more.
Any project that gives data scientists real-world experience can be extremely useful for their careers.
Step 4: Complete formal data science training
Formal study remains the most efficient way to ‘professionalise’ your data science skills. Formal data science courses offer structured learning experiences that are curated to provide training in the most valuable competencies in the field. This, in turn, makes independent work—and the learning that comes from it—more efficient. The benefits of formal study also include access to a network of academics and fellow learners and the ability to show employers a recognised credential as evidence of their skills. This is particularly essential to set yourself apart as a true data scientist.
The UNSW Sydney’s flexible online data science program is an ideal way to gain the skills necessary to become a data scientist. The university’s postgraduate courses include graduate diploma, graduate certificate and master’s-level degree programs, providing options for learners who want a complete training program and those who desire a shorter course to augment their existing learning.
A data science education from the university includes training in the profession’s core competencies and most in-demand skills, including statistics and machine learning. Furthermore, the university’s courses include the ability to specialise in these and other skills, such as database systems, so that you can target your education to your particular career goals. Moreover, the university is internationally ranked as a top school for statistics, mathematics and computer science, and employers understand that its graduates have the skills they need to be successful in their fields.
Data scientist salary and job outlook
As members of a profession that’s in strong demand, aspiring data scientists can expect a promising job outlook and generous salaries as they move through their careers.
- Job outlook: The Australian government reports that positions for data scientists increased more than 600 per cent between 2015 and 2019, and demand is expected to continue. The IT recruitment agency Hays predicts that data science roles will grow by 11 per cent by 2024
- Average salary for a junior data analyst: According to Payscale, the average salary for a junior data analyst was AU$60,442 as of May 2023.
- Average salary for a data scientist: According to Payscale, the average data scientist salary is AU$93,820 as of August 2023.
- Average salary for a data scientist manager: According to Payscale, the average salary for a data scientist manager was AU$143,900 as of August 2023.
Take the next step in your data science career
Becoming a data scientist can be rewarding for a variety of reasons. It enables you to learn a diverse range of skills and offers the opportunity to do meaningful, interesting and impactful work.
Find out more about UNSW Online's Master of Data Science today by visiting our website.