Can you teach yourself to be a data scientist?
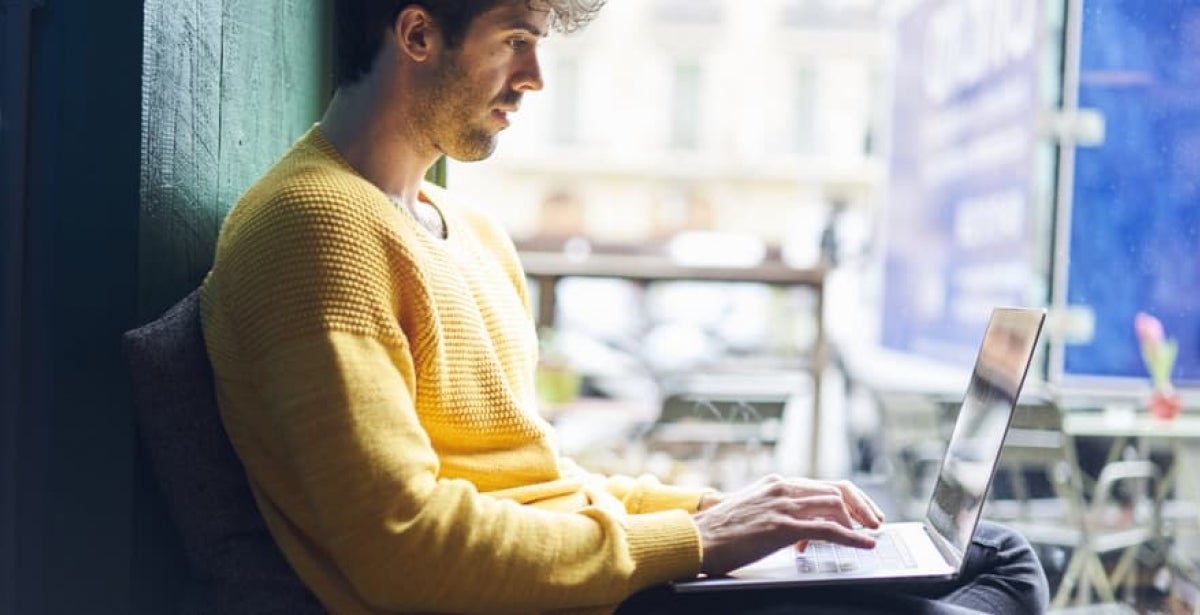
The number of education options available to would-be data scientists starting their careers has risen dramatically over recent years. Business and academia alike are seeking to take advantage of the rising demand for data science professionals, and have made effective use of innovations in online learning to do so. These learning options are roughly divided into two groups:
- The self-learning group which includes massive online courses (MOOCs) and short boot camps covering a range of topics such as programming, mathematics and machine learning topics.
- The formal education group which includes university and accredited professional programs such as UNSW's Master of Data Science.
Both options are useful, and today’s data science industry is comprised of professionals from both backgrounds. Because both paths can lead to success, the question of which path is more suitable for those seeking to become a data scientist is a matter of some debate.
There is no singularly correct answer as to which path is best. Self-taught learning is ideal for individuals who learn best with minimal structure, while the expert guidance that students receive through formal education offers the most assured path to professional success (75% of data scientists hold an advanced degree). The right choice depends on each student’s individual goals, priorities and learning styles.
Independent learning, a brief review
Self-directed learning is ideal for individuals that don’t thrive in formal education and work best when taking an exploratory approach to learning. Independent learning is also useful for individuals who want a basic introduction to data science before entering formal education.
The primary challenge of self-directed study is the lack of a clear pathway from beginner to professional. Individual MOOCs or boot camps that offer “all the things you need to get started” don’t offer enough education to enter the field, which means learners must figure out how to combine disparate learning resources into a comprehensive education. In order to become established members of the data science community, it’s necessary to invest significant time into activities such as networking and portfolio-building.
Key independent learner activities
Networking
To establish yourself as a trustworthy professional, it is vital to make yourself known to the wider data science community. This can be accomplished by actively participating in relevant social media discussions, meetup groups and Q&A sites such as Stack Overflow and Stack Exchange. Networking is a vital way to identify potential skill gaps, learn what skills are most in-demand and find opportunities for collaborating with others.
Community feedback
Self-learners don’t have the ability to rely on professor feedback, and must instead rely on community feedback to gauge their progress and learn what aspects of their craft they need to improve. This can be accomplished by taking steps such as posting data science projects to GitHub and discussing your work (conducting analysis) on blog sites like Medium. Many self-learning programs include basic instructions on using GitHub as a home for your data science portfolio.
Portfolio building
Many self-learners focus on practicing skills, not completing projects. However, potential employers need to know that their new hires can work in a real-world environment. By building a portfolio of independent data science projects, you can show employers that you have the initiative and problem solving skills necessary to succeed as a professional.
Reference building
The path from education to professional practice is less distinct for self-learners than it is for those in formal courses. While it is possible to simply keep expanding your portfolio until you find a full-time job, basic experience can be earned by looking for “gig work” as a freelancer or by collaborating with other data scientists. The people you collaborate with can become valuable professional references.
The additional advantages of formal learning
A formal education does not make networking, portfolio building or any of the other above practices redundant. Rather, a formal education stands apart from a self-guided process due to the robustness of its curriculum and the emphasis it places on quality control. The efficiency and structure of a formal education creates a learning journey that is more predictable and provides students with more opportunities to fulfil their goals.
Professional recognition
While self-taught learning requires learners to figure out how to build an attractive portfolio, degree-based learning programs are designed to produce job-ready portfolios as part of students’ journey through their course. Moreover, a data science degree provides graduates with a “foot in the door”, especially with the many large employers that consider formal certifications as part of their hiring criteria.
Built-in professional networks
Graduation from a degree program provides students with access to an alumni network comprised of fellow data scientists. These alumni are a useful source of advice, and can provide useful introductions to potential employers they have worked with.
Curriculum development
Curriculum development is a complex and time consuming process. While self-learners need to invest time developing their own curriculums, those in formal programs can rely on a curriculum that has been developed by experts who understand exactly what skills employers are looking for. Since students don’t need to spend time figuring out what to learn next, they can spend more time actually learning.
Program completeness
Because they need to develop their own curriculums, independent learners must also self-evaluate the completeness of their learning—whether they’ve learned “enough” about a given subject or still have gaps. In contrast, a data science degree is designed to provide the full range of skills necessary to start in the industry.
Professional guidance
Data science is a complex discipline, and learners in any situation can hit stumbling blocks which interrupt their progress. For self-learners, it can be difficult to figure out how to overcome these challenges. Students in formal programs benefit from ongoing access to peers, professors and advisors that can understand their curriculum and help them to decide on the next steps to take.
Scheduling
If you’ve ever found yourself not in the mood to work, but forced yourself to do so anyway because of an upcoming deadline, you are likely the type of person that benefits from the structure of a formal learning program. Formal programs provide a concrete path from start to finish, which means that there’s always an incentive to keep learning and never a question of whether you’re doing “enough” to succeed.
A formal education mitigates risk
The benefits listed above collectively mitigate the risk that time invested in a data science education won’t pay off. It is possible to become a professional data scientist through independent learning; however, the number of individuals who enrol in open-access data science programs is far higher than the number who then go on to find work in the field. Even authors of notable “teach yourself data science” guides have ended up working in adjacent fields instead of working as actual data scientists.
The higher success rates associated with formal education programs stems from the fact that these programs’ rely on their reputation for producing successful graduates in order to attract new students. For example, the University of New South Wales is ranked third in Australia for graduate employability. Students who enter UNSW’s data science program know that the school has a vested interest in ensuring that they graduate with the skills necessary to find work.
Commit to a future as a professional data scientist
The path to becoming a data scientist requires dedication and commitment. By providing students with a purpose-built curriculum and a predetermined path to graduation, formal learning programs maximise the likelihood that commitment will pay off. Self-taught learning opportunities are a wonderful resource for learners who don’t thrive in structured education programs; for those who do, formal learning programs can offer a significant competitive advantage.
The University of New South Wales’ online Master of Data Science program offers the best of both worlds, combining all the benefits of a formal education with the “learn when and where you want” flexibility of an independent study program. The program is designed to teach students the skills that employers value most, but also avoids taking a “one-size fits all approach” by offering students the ability to specialise in fields such as machine learning, database systems and statistics.
Featuring a curriculum developed and taught by experts with real-world experience, UNSW’s Master of Data Science offers a complete path to becoming a professional data scientist. As the only school in Australia that is top-ranked globally in math, statistics, computer science and economics, students in the program benefit from the expertise of professors who are among the thought leaders in their field.
If you want an education that combines the positive flexibility of independent study with the certainty and quality-control of a top-tier formal education, UNSW’s Master of Data Science offers an ideal path forward.
For more information about what UNSW’s data science program has to offer, contact our Enrolment team on 1300 974 990.