Top three most interesting data science projects of the 21st century
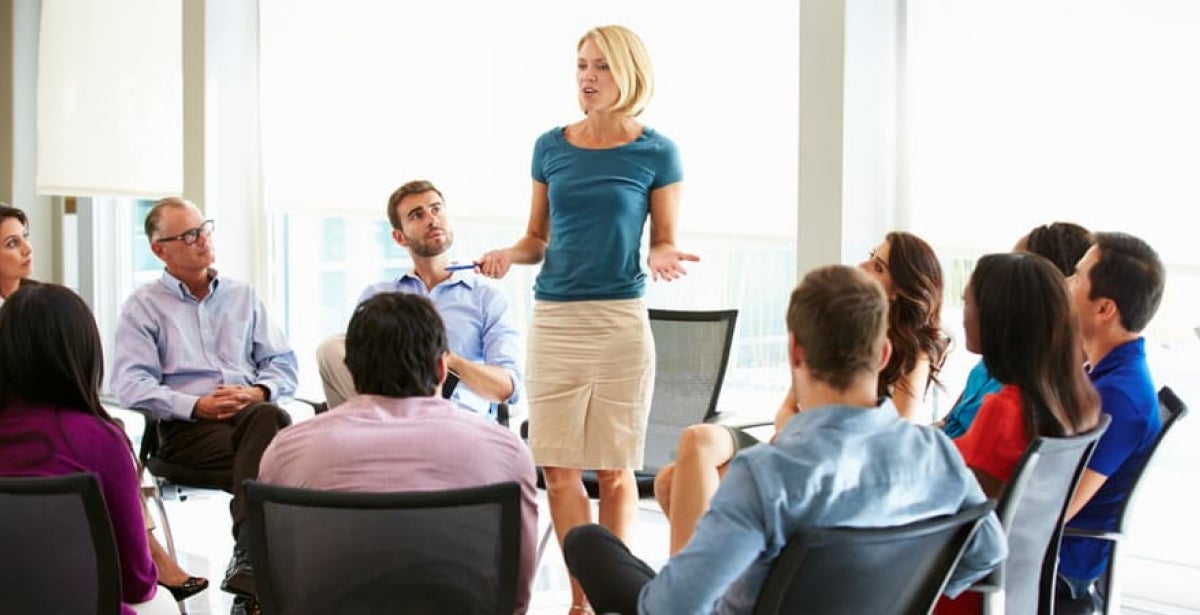
Data science is an incredibly flexible discipline that can be used to address a wide range of social and commercial challenges. Whether it’s molecular biology or astrophysics, structural engineering or poverty reduction, any problem that can be described with data can be examined and better understood through the work of data scientists.
The spread of data science throughout the economy is providing data scientists with the ability to work on a multitude of projects that make innovative use of their talents. Many of these projects are business-focused, while others rely on improving public services such as hospital access and firefighting. Across Australia and around the world, data science offers unparalleled opportunities for individuals who want to pursue exciting projects that make a difference.
Forecasting emergency room visits | Predictive modelling & healthcare
Healthcare is a significant area of focus in the data science industry. Data scientists specialise in figuring out how to make processes more efficient, and health care providers are constantly looking for new ways to maximise their budgets to improve the quality of care they can provide.
What did the project consist of?
A critical and interesting data science project tried to forecast emergency room visits. Australia’s rural health care districts operate on tight budgets, and many rely on resource sharing between care centres to function. To ensure that those resources are always where they’re most needed, the government of New South Wales hired a private firm to investigate whether data science could be used to predict emergency room volumes at rural hospitals and clinics.
Impact on the data science industry
This project analyzed a significant amount of data. Accurate predictive modelling requires large volumes of information for data scientists to work with; the hospital involved in this project provided 10 years of electronic medical records, including visit, admissions and discharge data. This data was then enriched with additional data, such as weather data, that could be used to discover new predictive relationships, such as the impact of high temperatures on admissions rates.
Project results
This data science project had significant positive results. By integrating this information into a machine learning framework, the data scientists were able to successfully create a predictive model that could reliably forecast day-to-day variations in the volume of emergency room visits. Hospital administrators can use it to ensure that they have the resources to meet what would otherwise be unexpected spikes in the demand for emergency services.
Projects of a similar nature have been repeated since then, using similar models of statistical analysis. These projects have repeatedly been able to predict variation in visits, as well as unearthing other critical information, such as influential variables for infection rates, hospital safety and health care risk factors.
Explaining a healthcare crisis | Root cause analysis & epidemiology
When an unexpected disease outbreak occurs, governmental public health institutions find themselves in a race against time to discover the root cause of the outbreak. The US Center for Disease Control (CDC) faced this situation in 2014 when they detected an HIV outbreak in Scott County, Indiana (pop. 4500) that was ultimately found to have infected 215 people.
Scott County has a history of opioid addiction issues, but the HIV outbreak was a new problem.
What did the project consist of?
To fully understand what was happening, the CDC turned to data science. Countless variables can contribute to disease outbreaks, but the ability to programmatically analyse big data allowed key factors to be discovered quickly.
Initial work on this type of problem often requires the use of exploratory data analysis (EDA), which allows data scientists to quickly inspect datasets for significant information. For example, the use of a time-faceted correlation matrix could enable scientists to examine what factors most strongly correlate with newly infected persons at different stages of the outbreak.
Impact on the data science industry
This project involved unique data science approaches. Classical machine learning techniques, such as random forest models, provided scientists with an effective way to analyse the outbreak. By leveraging machine learning techniques, the team was able to identify several factors behind the infection’s spread, including the unexpectedly strong role of sexual transmission. These details allowed the team to develop a profile of high-risk individuals, which in turn, enabled the CDC to identify additional persons who might be unknowingly infected with the virus.
Project results
The data science project yielded impressive results. Modelling of data allowed scientists to realise that 90 per cent of the infected population had previously injected a specific opioid drug called Opana ER. Opana had recently been reformulated so that it couldn’t be snorted, only for opioid addicts to discover that the new formulation could be easily injected instead. The discovery of its key role in facilitating Scott County’s HIV outbreak led to the U.S. Food and Drug Administration (FDA) declaring it illegal for sale in the United States.
Predicting bush fires | Big data integration & environmental Science
Climate change has turned environmental fires into a growing concern throughout the world. In Australia, increases in the frequency of bushfires have made it vital for fire services to be able to predict when and where fires are most likely to occur.
What did the project consist of?
To develop a predictive model of bushfire incidence rates (time and location), academics working with Data 61 – the national government’s world-leading data science unit – mined massive environmental data (time, location, vegetation and so forth). By integrating that data with historical bushfire data, they were able to model bushfire incidence across the continent.
Impact on the data science industry
In this project, data science was a means to actually save lives. Developing a predictive model for bushfires required the researchers to account for multiple causal relationships that existed in the data – in other words, to figure out how much each variable increased or decreased the chance of a fire. To do this, the researchers used an ensemble deep learning approach that paired an unsupervised model (for modelling unknown relationships) with a supervised model (for modelling known relationships).
Deep learning methods are effective for this kind of work because they can deduce a dataset’s causal relationships more quickly than a human can, and work well in situations in which researchers are working with many variables. The bushfire researchers’ use of unsupervised deep learning models allowed them to define the problem, while their use of supervised learning models allowed them to solve it.
Project results
This project was critical for many reasons, and it yielded important results. This type of project has the potential to improve fire prevention measures, allow firefighting resources to be allocated more efficiently and decrease emergency response times. As the frequency of bushfires increases, data science-driven predictive forecasting could be important in limiting the harm that fires cause to life and property. In February 2021, CSIRO’s Data 61 partnered with the National Council for Fire and Emergency Services (AFAC) to develop a national-consistent bushfire prediction model.
Prepare to pursue passion projects as a data science professional
Data science is being used for a variety of innovative projects throughout Australia, including locating humanitarian hot spots, protecting vulnerable children, and using computer vision to facilitate pain management. Some of these projects are commercial, others are non-profit, and still others are academic—data science projects permeate all sectors of the economy. A New Zealand company even created a program to perform facial recognition on sheep.
Data science is an excellent career choice for anyone seeking a high-demand job that offers the opportunity to work on something they’re passionate about. UNSW’s online Master of Data Science program provides an ideal way to enter the field.
The Master of Data Science is designed to ensure that students graduate job-ready, and it also offers the ability to specialise in specific areas of interest such as data engineering, statistics, and machine learning.
To learn more about how you can establish yourself as part of one of the world’s most in-demand professions, contact our enrolment team on 1300 974 990.