The democratisation of Artificial Intelligence
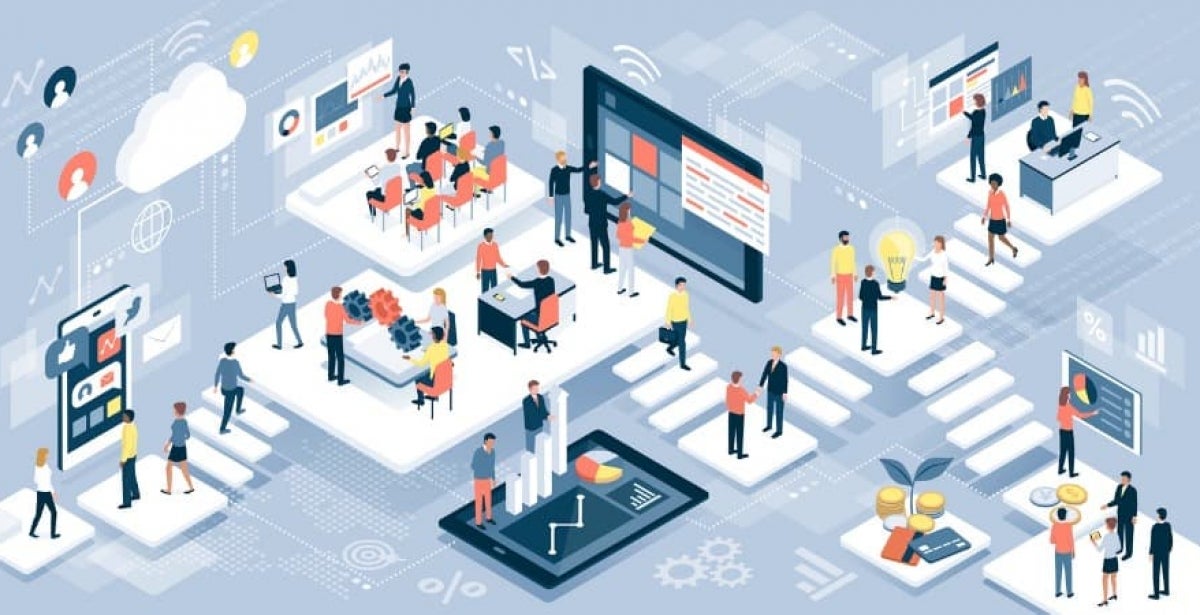
The ongoing development of artificial intelligence technology is transforming the global economy and creating new opportunities for innovation. However, because only a relatively small number of people possess the skills necessary to develop AI programs, there is a growing movement to make the technology easier to use and more accessible to a general population. This initiative to make AI more accessible is commonly referred to as the “democratisation of artificial intelligence”.
The rationale for democratising AI
The push to democratise artificial intelligence is partially the result of the global data science talent shortage, which is increasing sharply as growth in demand outstrips growth in supply. That widening skills gap has created an incentive for tech giants to push the democratisation of AI as a means to enable laypersons to perform basic AI work without formal training. This would allow data scientists to focus on complex tasks while simpler tasks could be performed by others.
The democratisation of AI may also present a means of mitigating the negative impact of AI-linked job losses.
According to a report by PwC, artificial intelligence will have made 30-40% of jobs redundant by 2030. A study by Finder.com.au, based on data from an Oxford study, predicted that nearly 45% of Australian jobs were threatened by automation.
Democratisation advocates argue that democratising AI would maximize the value of AI by ensuring that as many people as possible can use it to innovate and contribute to the economy. This increased economic output could then be used to create new opportunities and benefits to offset the damage of jobs being lost to automation. It would also help prevent the tech giants that currently possess most of the world’s AI talent from continuing to accrue a disproportionate amount of the economic benefits of AI initiatives.
The move to democratise the accessibility of artificial intelligence tools could also limit potentially negative effects related to the misuse of technology. Artificial intelligence programs draw conclusions based on the data they’ve been given to learn from, which means that they automatically reproduce—and potentially exacerbate—any biases that exist in the data. By increasing the number and diversity of people using AI, it is less likely that biased programs will be able to compete against the more representative programs that emerge within the AI marketplace.
Big tech's efforts to democratise AI
The democratisation of AI is fundamentally concerned with increasing the accessibility of AI technology at all levels of data science skill. Steps being taken to achieve this increase include making AI accessible to non-data scientists, encouraging the development of more non-professional “citizen” data scientists who work in AI and increasing both the number of professional data scientists and the number of new companies working in data science.
A number of large tech firms have taken steps to democratise AI, both by making it easier to utilise and by increasing the number of people working to build their AI skills. For instance, in 2018, Google acquired Kaggle, a website that holds machine learning competitions, in order to promote data science education. Moreover, large tech companies including Google, Microsoft and NVidia—whose graphics processors power deep learning AI applications—have poured millions of dollars into funding AI startups.
AI startups play an important role in the democratisation of artificial intelligence. Startup companies rely on innovating to succeed, and many of them are focused on making AI technology more accessible. Bonsai, a startup funded and later acquired by Microsoft, is working on projects dedicated to making the popular Tensorflow deep learning library easier to use. H20.ai, a startup funded by NVidia, provides an “AI automation platform” that is already used by over 5,000 different companies.
Tech giants are also developing AI democratisation tools in-house. For instance, Google describes its AutoML product line as “a suite of machine learning products that enables developers with limited machine learning expertise to train high-quality models specific to their business needs”.
Microsoft developed Windows as an easy-to-use abstraction layer for its text-based MS-DOS operating system.
The development of abstraction layers is a necessary part of making any technology accessible enough to achieve widespread adoption. Without an abstraction layer to make a technology generally accessible, its barriers to entry will be too high for the technology to become mainstream. For AI, the maintenance of these barriers would ensure the continuation of the current status quo, in which only a small part of the population has the ability to take advantage of the massive power that the technology offers.
Many popular data science tools, such as RStudio, already provide limited abstraction functionality to make the coding process more efficient. AI democratisation software takes things a step further by making the development process easier and less reliant on technical knowledge. This is accomplished by either reducing the complexity of the AI programming process (low code tools) or automating aspects of it (no code tools).
The impact of democratising AI
The invention of the printing press is an early example of the positive impact of democratisation. The printing press made literacy more accessible, which in turn allowed the highly literate class to leverage the benefits of a more knowledgeable society into significant innovations in accounting, math, and science. Similarly, the expansion of AI tools to a low-skilled population will create a larger AI marketplace that professional data scientists can leverage to foster further innovation within their field.
The simplification of any technology does impose some limits on what that technology can do effectively. This means that advanced AI tasks will continue to require trained data scientists to grapple with the full complexity the technology has to offer. By reducing the AI accessibility gap, data scientists will have more opportunity to focus on these more complex tasks while lower-level work is handled by others. This is important, as the growing integration of AI into the economy will increase the amount of complex work that needs to be done.
Democratising AI requires data scientists
Just as growth in the size and number of internet networks has lead to increased demand for network professionals capable of managing the resulting growth in network complexity, growth in the number of AI applications will increase demand for skilled data scientists. Democratisation will make AI development more accessible; however, improved accessibility will not preclude the need for individuals that possess the ability to consider technical matters, such as the impact that data quality and choice of statistical model have on the effectiveness of a given AI.
Professional data scientists understand the importance of these technical details. As non-experts begin to create AI products and services without knowing how they function “under the hood”, it will fall to them to ensure that those products and services are both safe and effective.
The University of New South Wales’ Master of Data Science program offers an ideal way to build the skills necessary to leverage the opportunities—and confront the challenges—of AI democratisation. The university’s flexible online programs include masters, graduate diploma, and graduate certificate courses, all of which place heavy emphasis on teaching the machine learning concepts that underpin modern AI technology. UNSW is also globally ranked as a top school for economics, mathematics, statistics, and computer science—the core subjects of a data science education. As the role of AI in society continues to develop rapidly, there is no better way to get started in this fast-evolving field than through education at UNSW.