How to transition from a data analyst to a data scientist
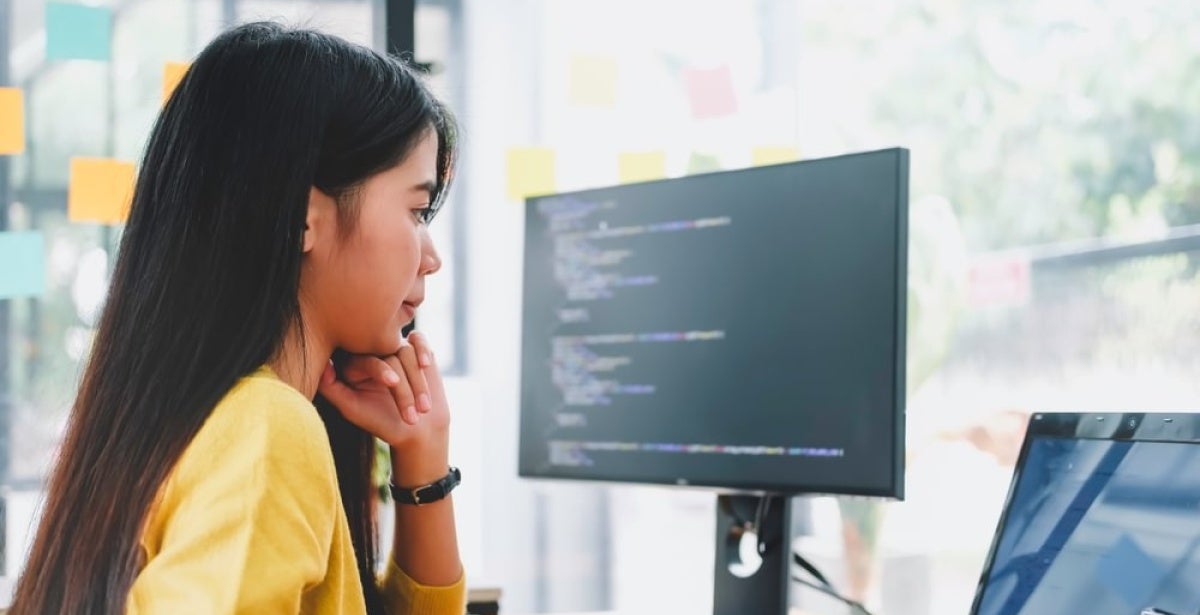
Today, as the job market continues to shift, many professionals are changing roles and retraining for careers that are ready for the 21st-century economy.
One industry that’s particularly in demand is data science, thanks to the rise of big data, machine learning and artificial intelligence. However, skills shortages still exist, and many professionals lack the training to fill these positions in Australia.
This means that data analysts have a unique advantage in accessing this in-demand industry. Although data analysts and data scientists have distinct roles and use different methodologies to achieve their goals, both focus on leveraging empirical data to create value for their businesses.
This synergy makes data science an ideal field for data analysts who want to transition to a career that will allow them to use the skills they’ve learned in their current role.
Below, we’ll explore what you need to know about transferable skills, tips for transitioning, and the educational and professional steps to becoming a data scientist.
Why transition from data analyst to data scientist?
There are many compelling reasons for a data analyst to want to transition into the role of a data scientist. Here are three.
Stay on the industry’s cutting edge
The growing importance of data science means that professionals in the field are increasingly sought after. Transitioning from a data analyst to a data scientist role allows professionals to remain at the forefront of the industry, engaging with—and even developing—the latest technologies and methodologies.
As more businesses look to leverage data-driven insights, data scientists are in a prime position to use advanced analytics to deliver greater value.
Enhance technical expertise and career opportunities
Becoming a data scientist provides an opportunity to master sophisticated techniques in areas such as machine learning, natural language processing and neural networks. This expertise sets these professionals apart and enables them to work on more complex and impactful projects.
Transitioning to a data science role can open the door to a broader range of career opportunities as an invaluable asset to organisations aiming to harness advanced analytics.
Influence business strategy and drive value
A data scientist’s unique capabilities, such as being able to model and structure data from millions of data points, are instrumental in helping businesses make strategic decisions. Tools such as predictive modelling and machine learning enable a level of accuracy and efficiency that goes beyond traditional data analysis.
Becoming a data scientist can mean playing a key role in shaping future business strategies and making data-driven decisions that contribute significantly to an organisation’s success.
What transferable skills do data analysts have?
A worker’s ability to change careers is a sign of their competitiveness within the labour force. For data analysts looking to further their career through a transition into data science, their existing career experience provides them with a major competitive advantage within their new field.
Key skills
The data analyst skill set includes competencies that employers value but that many data scientists currently lack, such as industry-specific business knowledge and the ability to effectively communicate their insights to key decision-makers.
Data analysts have numerous skills that are easily transferable to a role as a data scientist, including the following:
- Ability to interpret data and translate it into business solutions
- Understanding of how to design data collection and analysis methods and processes
- Knowledge of how to identify patterns and trends in data
- Understanding of data analysis software
- Ability to develop, implement and maintain data infrastructure
- Understanding of how to create data visualisations and reports
Ability to work in a variety of industries
Many data scientists work in a manner that is relatively industry-agnostic. While this means that data scientists can use their skills in a variety of different business environments, it also means they may not understand the “on-the-ground” needs of their employer.
This may limit the data scientist’s ability to turn their work into an actionable source of value for their employer, an issue that contributes to why some companies are unable to generate value from their data science programs.
A data analyst’s industry-specific expertise provides them with the ability to understand any operational or policy obstacles that may impact the usefulness of their insights. Data analysts-turned-data scientists stand out among data scientists due to their ability to recognise the practical needs of employers who operate within the industry that they are experienced in.
Communication
The communication skills that data analysts must hone throughout their careers are another major competitive advantage in the data science world. Soft skills are extremely important for data scientists, who must be able to clearly communicate their insights to company decision-makers who do not possess significant data literacy.
As many data scientists currently lack these skills, a data analyst who can demonstrate their soft skills will be well-positioned to succeed as a data scientist.
What skills do data analysts need to learn to become data scientists?
Although data analysts and data scientists both focus on maximising the value that a business can get from its available resources, the methodologies they use to do so differ significantly, which is important for data analysts to understand before they become data scientists. A move into data science requires data analysts to learn the data modelling and analytics skills that data scientists rely on.
The following are the key areas that data analysts must gain skills in to make the transition successfully.
Coding
Modern data science relies heavily on coding tools to model and analyse data, which means that any individual transitioning into data science needs to learn how to code.
The most popular coding language used in data science is Python, and R is also used heavily. R is a data science-focused language, but Python is a general-purpose language, which means that Python learners need to ensure that they study it in a manner that emphasises its data science applications.
Maths and statistics
Data scientists rely on mathematical modelling to create insights from data; in fact, a key part of the data science learning process involves developing an intuitive understanding of what statistical tools to use to draw insights from a given dataset.
The data science learning process should include an overview of statistical techniques, such as linear regression and a basic study of algorithms, which are vital to predictive analytics.
Data organisation and preparation
Data scientists spend a considerable amount of their time on data preparation—basic tasks that include finding, manipulating and organising data, as well as exploratory data analysis (EDA). EDA includes the use of skills such as data visualisation, which data scientists use to better understand their datasets.
Because so much of a data scientist’s job is devoted to this type of work, it is necessary to learn how to do it efficiently by incorporating it into any training regimen.
Projects
One of the most effective ways for data analysts to demonstrate (and build) their capacity to work as data scientists is to complete projects to show potential employers. This may be accomplished through a number of means, such as asking their employer to do data science work as a form of professional development or starting an independent data science project in their spare time.
Additionally, many formal data science courses include project-based learning modules that can be included in professional data science portfolios upon their completion.
Tips for transitioning from a data analyst to a data scientist
As with any career change, transitioning from one role to another can be challenging. However, if you wish to become a data scientist, you should consider doing the following.
Invest in your education
Pursuing formal education is an excellent way to ease your transition from a data analyst to a data scientist role.
These programs offer curated, structured learning experiences designed to train you in the most valuable competencies within the field. Additionally, these programs offer access to a network of experts and fellow students and the ability to show employers a recognised credential on your resume.
UNSW’s Master of Data Science provides a structured path for data analysts to advance their careers by bridging the skills gap between data analysis and data science. The program covers crucial areas such as machine learning, data engineering and complex statistical modelling, empowering you to transition from descriptive analytics to predictive and prescriptive modelling.
Another valuable element of the program is that it emphasises real-world applications, using hands-on projects that simulate industry scenarios. This, in turn, prepares you for challenges a data scientist typically encounters.
Apply for a skilled volunteer position
If you’re a data analyst, you already have many transferable skills that you’ll need as a data scientist.
However, what you may not have is formal experience in the role. A great way to obtain this is to apply for a skilled volunteering position in this field. This involves volunteering for a nonprofit organisation that may be aligned with causes you care about.
There are many websites that offer skilled volunteering positions, such as SEEK Volunteer and Pro Bono Australia.
Complete an internship
Another option for getting formal experience as a data scientist is an internship. Internships may be paid or unpaid and usually involve completing entry-level tasks to obtain experience. After completing an internship, your host organisation may offer you a permanent role.
Seek a secondment or job shadowing opportunity
If you work in a large organisation that already employs data scientists, a great way to make the transition is to job shadow or undertake a secondment.
Job shadowing involves following an experienced data scientist to learn what tasks the data scientist undertakes and how to undertake them.
Secondments involve you actually making the switch to a data scientist role and learning on the job. After your secondment, which usually lasts for at least a few months, you may be offered a permanent position.
Develop a strong portfolio
A robust portfolio can showcase past projects that highlight your data science skills, such as your ability to create machine learning models, perform advanced statistical analyses, or work with unstructured data. Contributing to open-source projects or participating in data competitions like Kaggle’s can also strengthen your profile.
Your projects need to be documented clearly, detailing your problem-solving approach, data preprocessing techniques and model evaluations. A well-documented portfolio demonstrates your capability to tackle real-world data challenges and gives potential employers insight into your technical and analytical expertise.
Strengthen your technical proficiency
You can enhance your technical skills by mastering programming languages and frameworks that are essential in data science roles, such as Python, R, TensorFlow and PyTorch. You can also focus on cloud platforms like AWS, Azure and Google Cloud to better understand how to deploy models and work with big data solutions.
Enhancing your SQL skills and learning about data engineering concepts can also help you understand data pipelines and infrastructure. Staying updated with the latest tools and advancements keeps you competitive and equips you for various data science roles.
Power your career transition through a formal data science education
If you are looking to transition from a data analyst to a data science career, one of the most efficient ways to do so is through a formal education program, such as one of those offered by the University of New South Wales (UNSW).
UNSW Online’s Master of Data Science program offers working professionals the ability to learn data science flexibly, without disrupting their careers. This program equips you with comprehensive, in-demand foundational skills, as well as the opportunity to specialise in areas like machine learning, database systems, or statistics based on your career goals.
Data science is an in-demand field that offers high pay and strong employability. Get the skills and knowledge you need to transform your career path and make a successful transition into the field with UNSW Online.
To learn more about UNSW’s Master of Data Science, get in touch with our Enrolment team on 1300 974 990.